This is a summary of an episode of Pioneers, an educational podcast on AI led by our founder. Join 2,000+ business leaders and AI enthusiasts and be the first to know when new episodes go live. Subscribe to our newsletter here.
TL;DR
- The competitive landscape for artificial intelligence is shifting away from a focus on algorithms and infrastructure and towards access to unique, high-quality data.
- The commoditization of AI algorithms and infrastructure has made it easier for companies to get started but harder to maintain a competitive edge based on algorithms alone.
- Industry-specific data holds immense potential for developing powerful, differentiated AI applications that drive real business value.
- Acquiring and leveraging industry-specific data comes with challenges, such as the proprietary nature of datasets, data quality issues, and more.
- Collaborations between data owners (industry domain experts) and AI providers can unlock the full potential of AI in specific sectors.
Check out our full episode here:
Cory Jansen — Artificial Intelligence Pioneer and Serial Entrepreneur
Cory Jansen, CEO and co-founder of AltaML, has a rich history in entrepreneurship and technology. He started his journey with Investopedia, a financial education website he co-founded during his university days. Investopedia's success, which eventually sold to Forbes, highlighted Cory's ability to identify market gaps and create valuable digital products.
In 2015, Cory's interest in AI grew while addressing high content creation costs. He explored algorithmic content generation, leading to the creation of AltaML. AltaML focuses on applying AI to solve business problems and elevate human potential.
Cory’s goal is to bridge the gap between academic research and practical business applications by ensuring that AI's benefits are tangible and widely accessible.
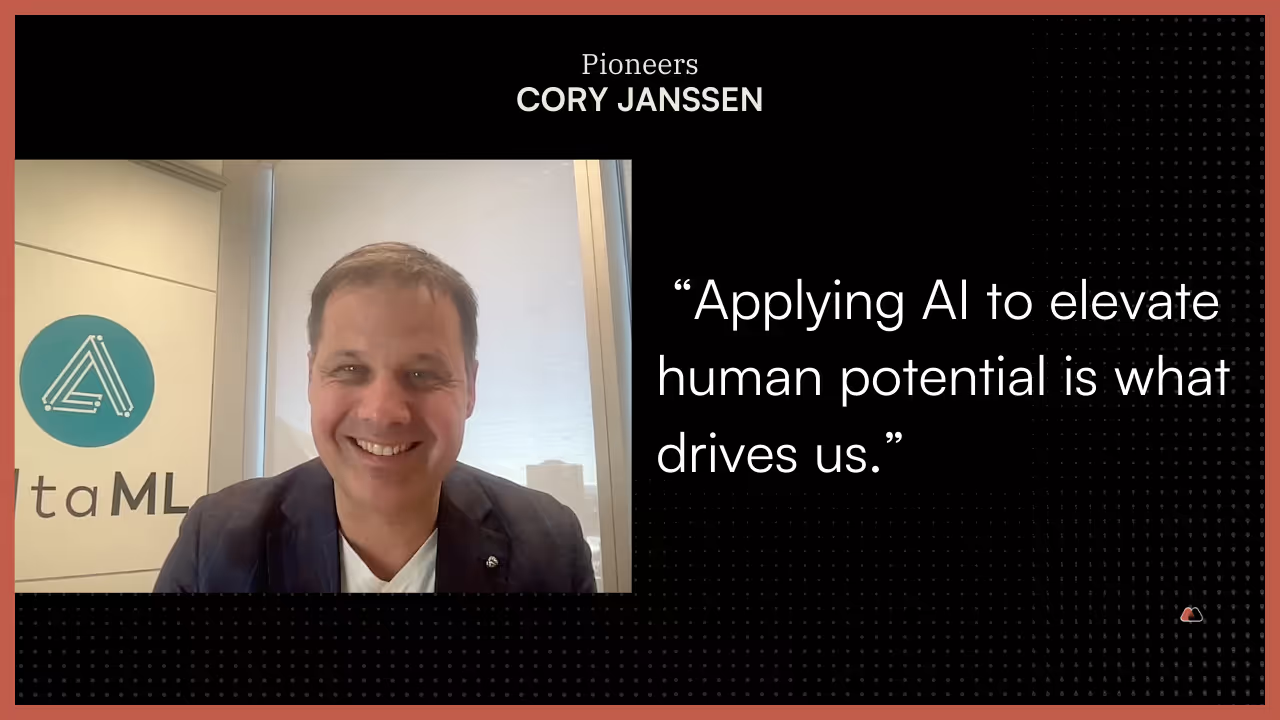
AltaML's mission is to enhance decision-making and operational efficiency across healthcare and clean technology industries. His approach combines strategic insight with a commitment to ethical AI use, positioning Cory Jansen as a significant influencer in the tech landscape.
AI Data Quality Is the New Competitive Advantage in Diverse Industries
As AI technologies become more advanced and widely adopted, companies are racing to harness their potential to gain a competitive edge.
From healthcare and finance to manufacturing and retail, AI is being applied across various industries to optimize processes, improve decision-making, and drive innovation.
In recent years, there has been a surge in investment in AI, with companies pouring billions of dollars into developing cutting-edge algorithms and infrastructure. This investment has led to rapid advancements in AI capabilities, making it easier for companies to leverage AI to solve complex problems and drive business value.
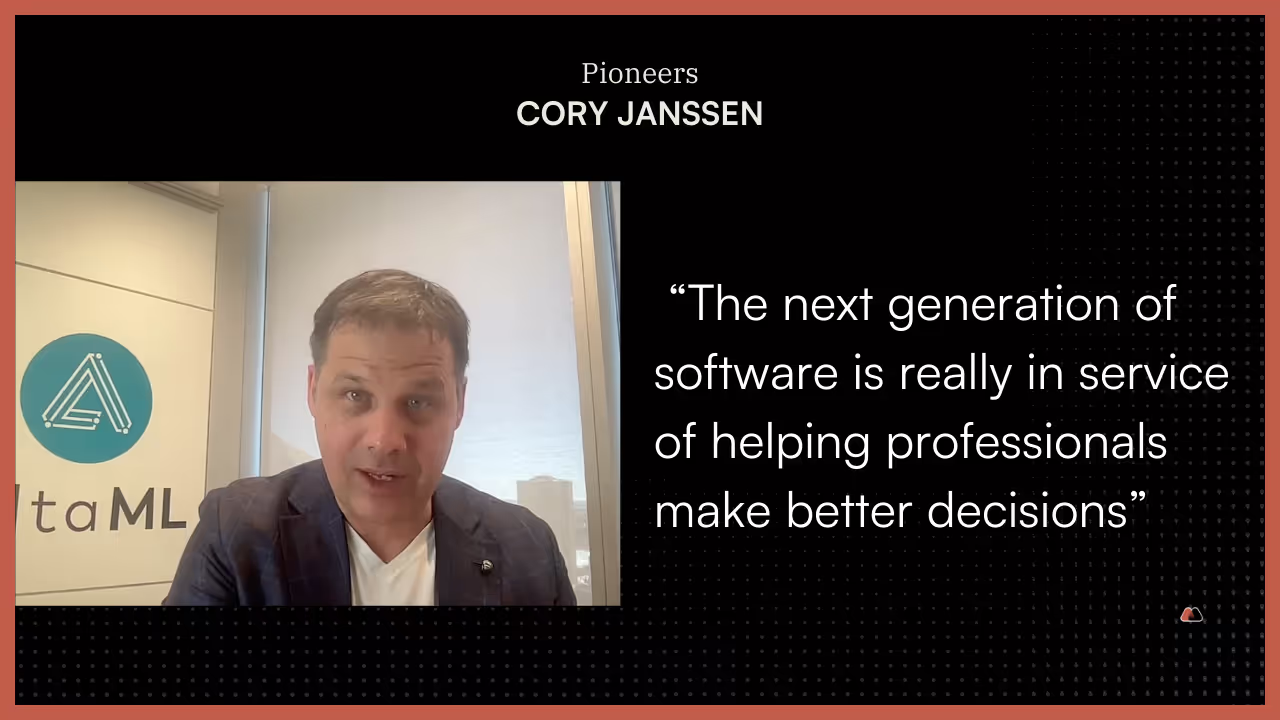
But as the AI industry matures, the competitive landscape is shifting. While having access to state-of-the-art algorithms and infrastructure remains important, it is no longer the sole differentiator for success.
As Cory points out in our podcast interview, data quality and machine learning hold the key to success and outsized advantages. Access to unique, high-quality, accurate data will be the key differentiator for AI success, not algorithms.
As AI algorithms become more commoditized and accessible, the competitive advantage will shift.
Companies that possess data quality tools tailored to their specific industries and know how to define data quality standards will be the winners in this game.
When combined with domain expertise and effective AI strategies, AI data quality will enable companies to build powerful, differentiated AI applications that drive real market value.
Enhancing Business Value with AI Algorithms and Infrastructure
In recent years, we’ve witnessed a significant shift towards the commoditization of AI algorithms and infrastructure. What was once the domain of a few tech giants and research institutions is now becoming increasingly accessible to a wider range of companies.

The open-source movement drives this trend by making many cutting-edge AI algorithms freely available. Additionally, cloud computing democratizes access to powerful computing resources, enhancing data management practices across various industries.
This highlights how quickly the AI landscape is evolving, driving innovation and how easily algorithmic advantages can be eroded.
Hyperscalers
Another major factor in the commoditization of AI is the role of hyperscalers like Amazon Web Services (AWS), Google Cloud Platform (GCP), and Microsoft Azure. These cloud computing giants have made significant investments in AI infrastructure, offering a wide range of services and tools that make it easier for companies to build, train, and deploy AI models at scale.
“A stat that not a lot of people know about is that over 80% of the flow in 2023 into AI was to seven companies.“ — Cory Jansen
He says hyperscalers have lowered the barriers to entry for companies looking to leverage AI by providing access to powerful computing resources and pre-built AI services.
Competing on Algorithms and Infrastructure Alone Isn’t Viable
While the commoditization of AI algorithms and infrastructure has made it easier for companies to get started with AI, it has also made it harder to maintain a competitive edge based on algorithms alone.
Instead, the real differentiator will be accessing and leveraging unique, high-quality data to train industry-specific models.
"There's datasets that don't exist on the internet. So unless you have a deep understanding of the workflow and unique edges on data, you're not going to have solutions that work in that industry." — Cory Jansen
As the AI landscape continues to evolve, the focus will shift towards data as the key differentiator, with companies that can access and leverage proprietary datasets being best positioned to succeed.
Data Quality and Machine Learning
Companies that acquire, manage, and leverage proprietary datasets tailored to their specific domains will be well-positioned to build powerful, differentiated AI applications that drive ROI.
One of the key advantages of industry-specific data is its ability to capture the unique nuances, challenges, and opportunities within a particular sector.
“If the data is not on the internet, there are unique edges on it that can be built from not just private datasets, but how users are working and utilizing that," — Cory Jansen
Cory emphasizes that companies can develop an AI model that is fine-tuned to their industry's specific needs using niche datasets. This enables insights and improvements that generic datasets cannot achieve.
- In healthcare, leveraging AI and predictive analytics with proprietary patient data can develop diagnostic tools that identify diseases earlier and more accurately.
- Financial sector companies can analyze large volumes of unique consumer behavior and transaction patterns to build AI models for fraud detection, risk assessment, and personalized investment recommendations.
However, acquiring and utilizing industry-specific data presents challenges.
Its proprietary nature and potentially poor data quality require significant preprocessing and cleaning. Companies that navigate these challenges and build robust data pipelines will capitalize on the untapped potential of industry-specific data.
Machine Learning Algorithms and Hallucinations
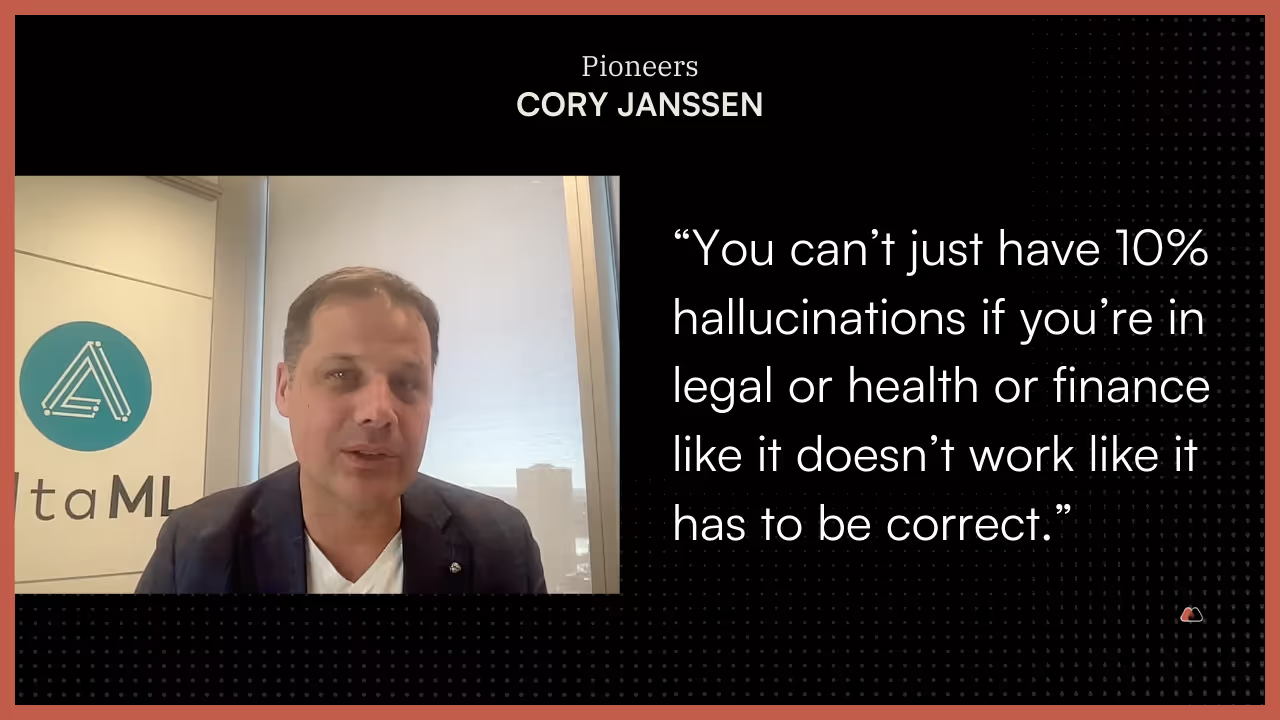
During the podcast, Ankur and Cory touched upon another issue with large language models (LLMs) known as hallucinations. While LLMs have significantly transformed how we communicate with software, Cory explained that they are prone to generating inaccurate information.
This flaw is particularly problematic in high-stakes industries like legal, health, or finance, where accuracy is paramount.
For AI to be reliable in these sectors, he says that integrating a robust data governance framework and employing data quality management processes is essential. Utilizing tools such as knowledge graphs or other sources of truth ensures data accuracy and mitigates the risk of hallucinations.
Ensuring AI Data Quality Through Collaboration with AI Providers
As AI evolves, the focus on industry-specific data as a key differentiator highlights the need for collaborations between data owners and AI providers. These partnerships combine domain expertise with technical know-how, unlocking AI's potential in specific sectors.
Data owners possess valuable proprietary datasets but may lack data quality metrics and data cleansing tools. AI providers with expertise in machine learning algorithms and data quality management can assist in ensuring data quality standards.

Cory emphasizes the importance of bridging this gap. Collaborations enable data owners to leverage AI providers' expertise in data preparation, data enrichment, and deployment strategies.
This partnership fosters the development of powerful, industry-specific AI applications. It also promotes innovation and continuous improvement, breaking down silos and creating growth opportunities through new business models and revenue streams.
The Future of Machine Learning and Data Quality
As the AI landscape continues to evolve and mature, it is becoming increasingly clear that the most successful companies will be those that can effectively leverage industry-specific data to develop powerful, differentiated AI applications.
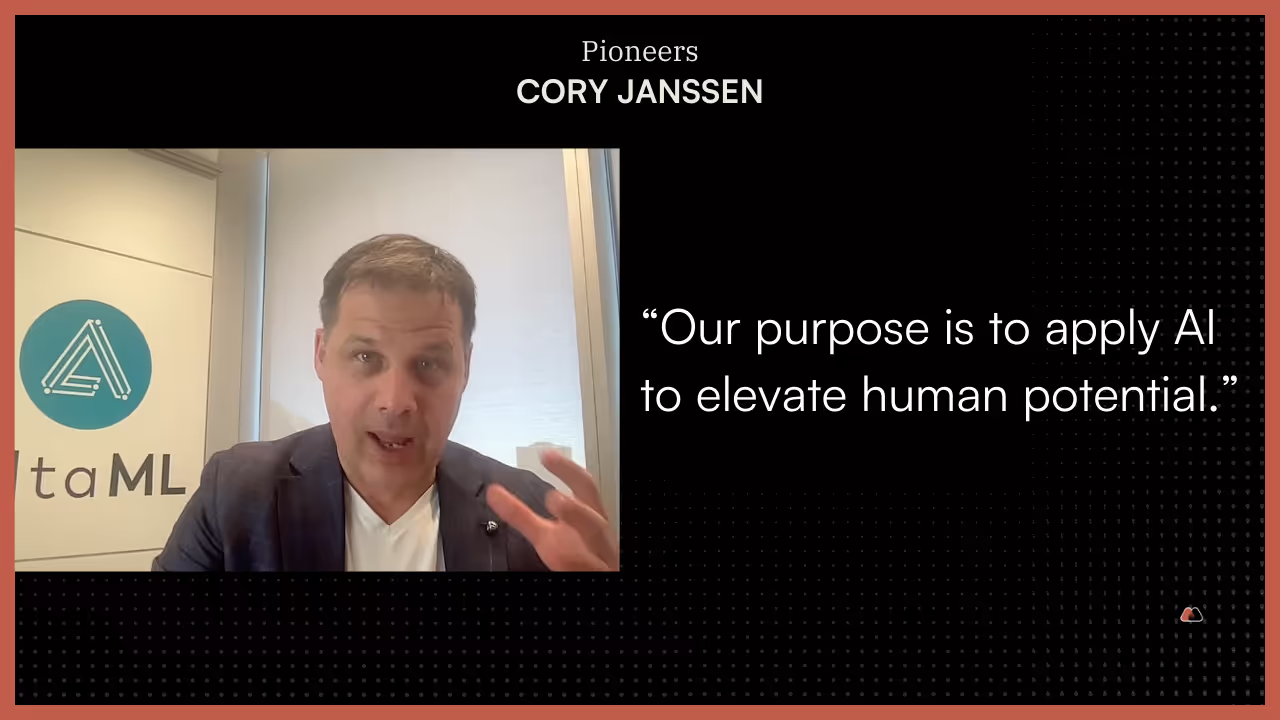
While having access to cutting-edge algorithms and infrastructure is certainly important, it is no longer enough to sustain a long-term competitive advantage. Instead, the future of AI competition will be shaped by organizations that can acquire, manage, and leverage proprietary datasets to unlock new insights, drive efficiency gains, and create innovative products and services.
Companies must focus on acquiring and leveraging high-quality data to gain a competitive edge with AI. This involves forging partnerships with data providers, participating in data exchanges, or investing in internal data generation.
Ensuring data quality through robust data management practices and data quality processes is crucial. By resolving data quality issues and standardizing formats, businesses can capitalize on industry-specific data and drive transformative business outcomes.
Ultimately, the future of AI will be shaped by those companies that can effectively bridge the gap between cutting-edge technology and deep industry expertise.
"It is the greatest time to be an entrepreneur. If you're working in this space and you understand how to use it, it's like you've just been given a brand new tool for your toolbox." — Cory Jansen
By staying at the forefront of AI innovation while remaining grounded in the challenges of specific industries, companies can unlock AI's full potential and drive long-term success. The race is on to capture the value of industry-specific data, and those who can effectively navigate this new terrain will be well-positioned to lead the way in the AI-powered future.