Making quick and accurate decisions in industries like healthcare, insurance, and banking is becoming more complex and difficult. Traditional decision-making processes are slow and prone to errors, leading to workflow inefficiencies. Manual processes struggle to keep up with the huge volumes of data needed to make informed decisions.
One solution is to use generative artificial intelligence (AI), which uses advanced AI algorithms to analyze large amounts of data to help your business automate your decision-making workflows.
What is Automated Decision-Making?
Automated decision-making systems process data to identify patterns and make decisions based on predefined criteria or learned behaviors.
It works by following four steps:
- Data collection: Automated systems collect data from multiple sources. For example, in healthcare, this can include patient records and medical histories.
- Data analysis: Algorithms and models analyze the collected data to identify patterns, trends, and correlations. In banking, this may involve analyzing market trends and financial statements.
- Decision rules: Based on the analysis, the automated system applies predefined rules or models to make decisions. Insurance companies, for example, can use algorithms to assess risk and determine premiums.
- Action implementation: The final decision is implemented automatically, which could mean approving a loan, processing an insurance claim, or recommending a treatment plan in healthcare.
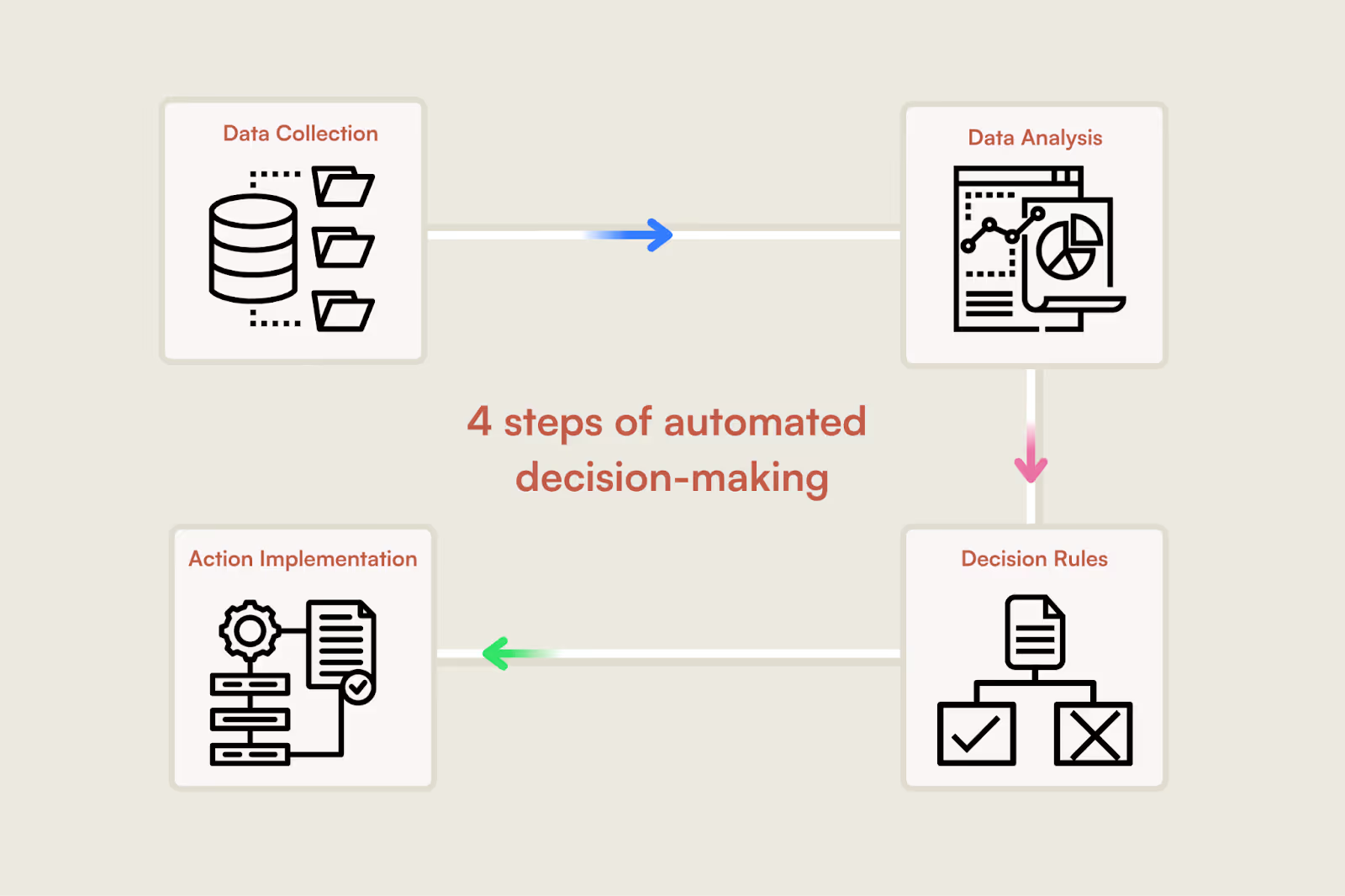
AI vs. Automation
Automation involves using technology to perform tasks without human involvement. Usually, it follows a set of predefined rules and logic to complete routine tasks efficiently.
In banking, automation involves automatically processing transactions, generating reports, or managing customer communications using predefined templates.
On the other hand, AI includes task execution and making decisions based on data analysis.
AI systems learn from data to help businesses make more informed decisions. Using the banking industry as an example again, AI can analyze market trends, predict stock movements, and make real-time investment decisions.
While automation is best suited for routine tasks with predictable outcomes, AI is better for handling complex tasks that require a deeper understanding and have uncertainty involved.
Decision Automation Types
Automated decision-making can be split into different types based on the methods and technologies used.
The two main types of decision automation are rule-based and data-driven, with each type having its own advantages and applications.
Rule-Based Decision Automation
Rule-based decision automation relies on predefined rules and logic to make decisions. These rules are created by experts who outline specific conditions and corresponding actions.
Some practical examples of rule-based decision automation include:
- Healthcare: Rule-based systems automate appointment scheduling by applying rules that consider doctor’s notes, patient preferences, and urgency of care.
- Insurance: Automated underwriting uses rule-based rules to evaluate insurance applications based on criteria like age, health status, and risk status.
- Banking: Financial businesses can use rule-based automation to process loan applications, making sure that only people who meet specific criteria are approved.
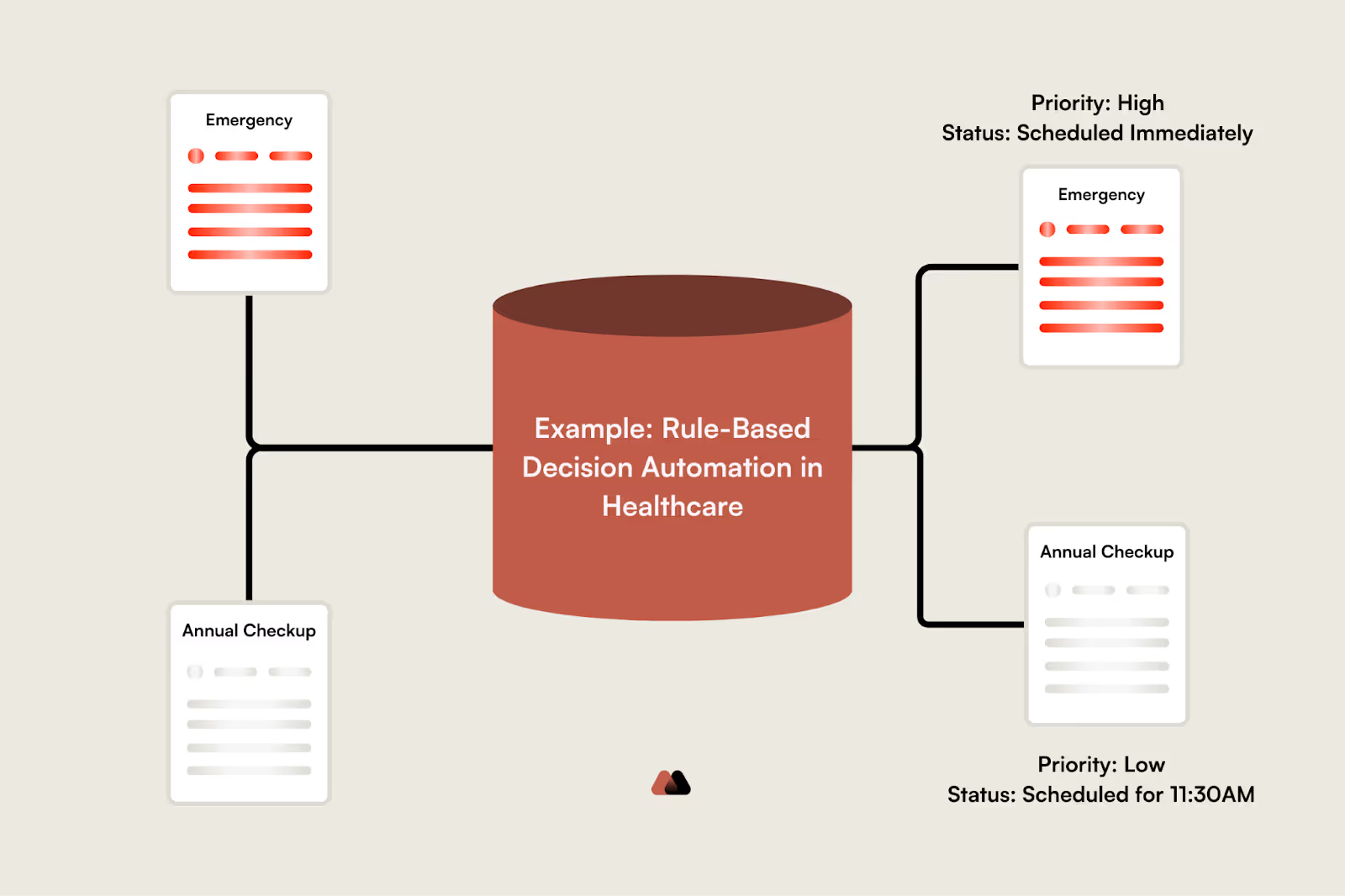
One advantage of rule-based decision automation is that it lets businesses make consistent decisions since they follow predefined rules. It’s also easy to implement for repetitive tasks.
However, this type of decision automation isn’t flexible, so it won’t adapt to new or unexpected situations. Moreover, it requires continuous updates as conditions change.
Data-Driven Decision Automation
Data-driven decision automation uses data analysis and predictive models to make decisions. Unlike rule-based systems, data-driven automation can learn from data and adapt over time, providing businesses with more flexible decision-making capabilities.
Applications of data-driven decision automation include:
- Healthcare: AI-powered diagnostic tools analyze medical images and patient data to assist doctors in diagnosing diseases effectively.
- Insurance: Predictive models assess the likelihood of claims and identify potential fraud, helping insurers make informed policy issuance and claims processing decisions
- Banking: Data-driven systems analyze market trends, customer behavior, and financial data to provide real-time investment recommendations and detect fraudulent transactions.
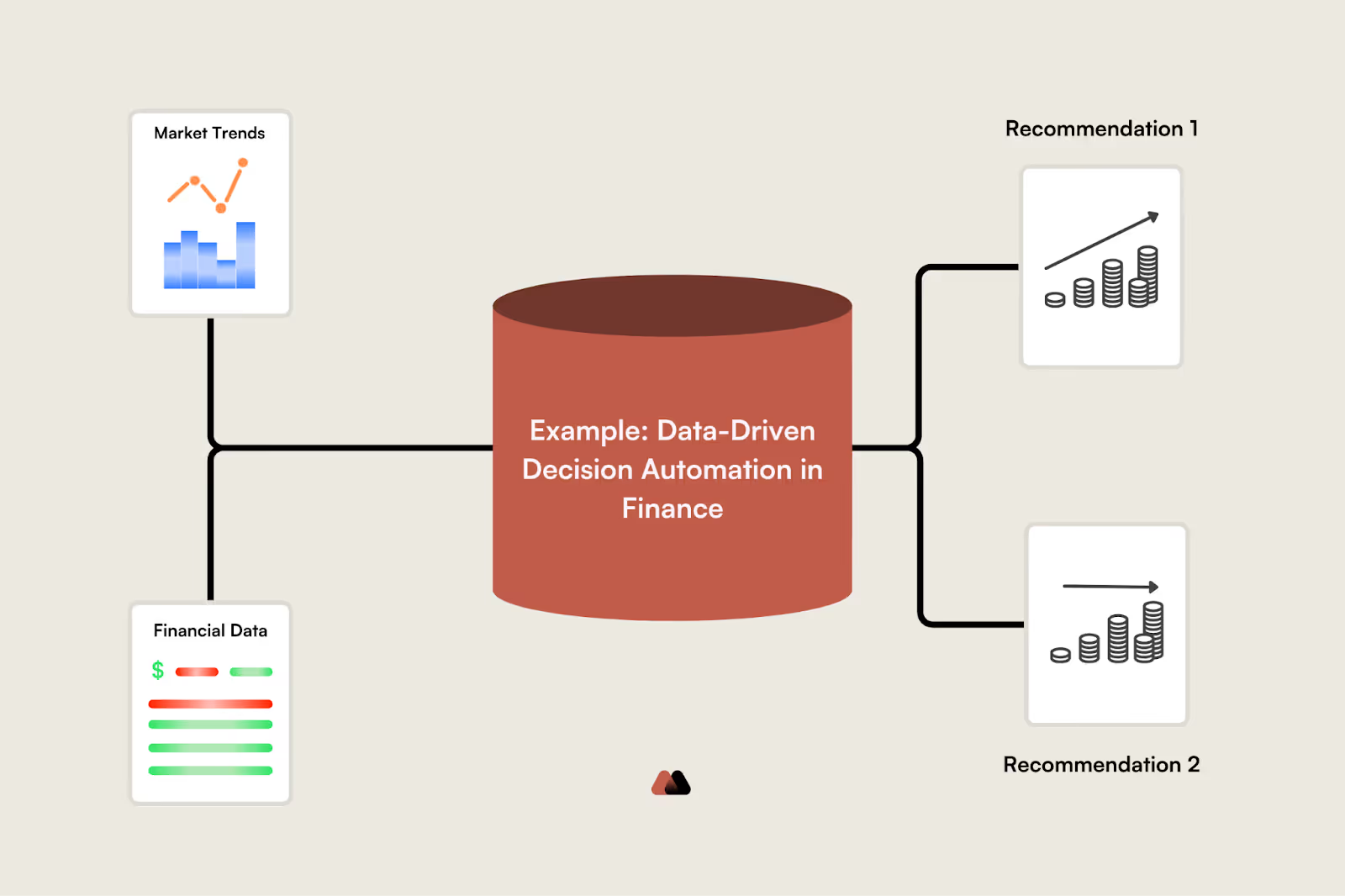
This type of decision automation can adapt to new data and evolving conditions, improving decision accuracy over time. Data-driven decision automation can handle large volumes of data and complex decision-making processes and provide deeper insights.
However, the output quality depends heavily on the quality of the input data. It also has a higher initial cost and a more complex implementation compared to rule-based systems.
3 Benefits of Decision Automation Over Manual Processes
Using decision automation has various advantages over manual processes. It lets businesses boost efficiency and accuracy while drastically reducing the chance of human oversight.
1. Efficiency
Manual decision-making is inherently slow—it involves human intervention at every step. Gathering data, analyzing information, and making decisions takes considerable time, resulting in delays and inefficiencies. Manually processing insurance claims, for example, involves significant time for data verification and approval, delaying customer claim settlements.
Automated decision-making processes are highly efficient by being able to process large quantities of data to make informed decisions, making it a much more time-effective option for businesses.
In healthcare, automated systems quickly analyze patient data and recommend treatment plans, reducing the time doctors spend on diagnosis so they can focus more on patient care.
2. Consistency
Human decision-making is highly prone to errors because of fatigue and oversight, leading to inconsistent outcomes and reduced reliability. Manual data entry in financial reporting can lead to inaccurate financial statements and compliance issues.
However, automated decision-making applies predefined rules and AI algorithms so that businesses can make accurate decisions. Automated loan approval systems apply the same criteria to all applications, which ensures fairness and consistency.
3. Scalability
Manual processes require significant human resources to manage increased workloads and volumes of data. Scaling up operations involves hiring and training additional staff - a time-consuming and costly process.
Scaling up manual claims processing requires more adjusters and administrative staff, resulting in higher operational costs.
Instead, automated decision-making processes can easily scale to handle increased workloads without needing a proportional increase in resources. Since it’s able to process large volumes of data simultaneously, it’s an ideal solution for businesses growing with expanding data needs.
Automated systems handle large volumes of patient data and diagnostics, helping healthcare businesses grow without expanding their teams.
Generative AI for Decision Automation
Generative AI uses algorithms like deep learning models to generate new data or content based on patterns learned from existing data. It goes a step further than simple automation by letting machines perform complex tasks that require a deeper understanding - making it highly suitable for decision automation.
It can process extensive datasets to recognize patterns and derive insights, helping businesses make informed decisions with an automated system.
Unlike traditional automation systems that rely on predefined rules and standard AI models that need human intervention for updates, generative AI continuously adapts and refines its decision-making processes based on new data. This benefits business environments where conditions and data are constantly changing.
In particular, AI Agents like ours use generative AI technologies to help businesses achieve full decision automation without requiring human intervention.
They automate various aspects of different workflows. For example, Decision AI automates decision-making workflows, and Document AI automates document processing workflows. Although each AI Agent is highly capable individually, they let businesses completely automate their workflows when working in tandem.
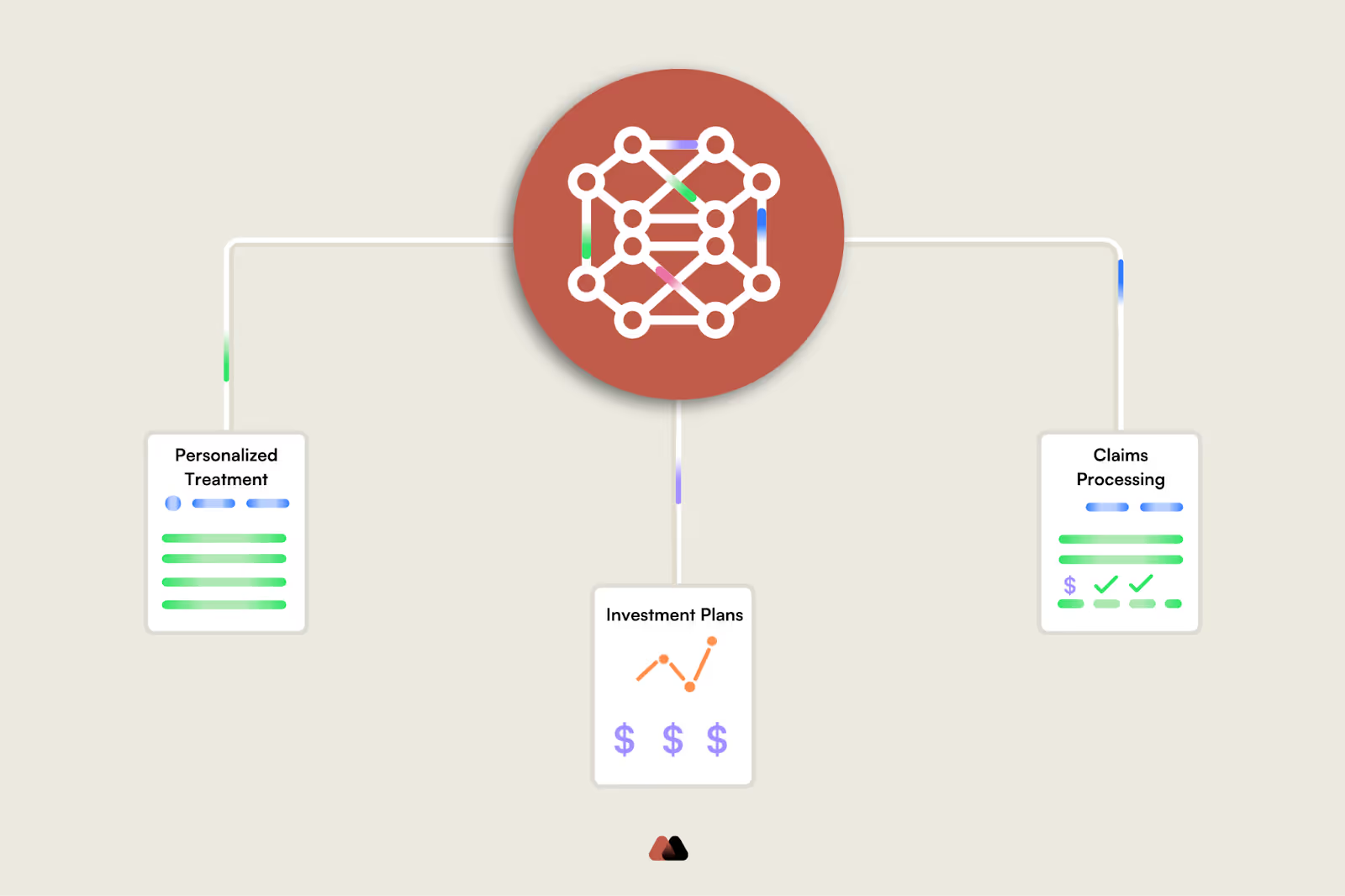
Why Use Generative AI?
Some key benefits of using generative AI for decision automation systems include:
- Proactive decision-making: Generative AI enables proactive decision-making by predicting future outcomes and trends. It lets businesses anticipate changes and make strategic decisions ahead of time instead of reacting to events as they occur.
- Enhanced personalization: Businesses can analyze individual data to offer highly personalized recommendations and solutions. For example, insurance companies can offer personalized policy options, while healthcare companies can develop personalized treatment plans at scale.
- Continuous improvement: Unlike static rule-based systems, Generative AI continuously learns and improves from new data inputs. This means businesses have a decision-making process that becomes more refined over time, increasing accuracy and reliability.
- Resource optimization: Generative AI optimizes resource allocation by efficiently analyzing and interpreting data to make informed decisions. Consequently, businesses can make better use of human and material resources.
- Full workflow automation: AI Agents use generative AI to take decision automation a step further by letting businesses fully automate their workflows. Other solutions only provide partial automation where much human intervention is still required, while AI Agents automatically provide instant decisions on key workflows.
Comparison With Other Automation Technologies
Generative AI has the upper hand compared to other automation technologies for various reasons.
Traditional Automation
As mentioned, traditional automation involves using technology to perform tasks based on predefined rules and workflows. These systems are designed to handle repetitive tasks efficiently but lack flexibility.
- Flexibility: Traditional automation is rigid, following strict rules and procedures. Generative AI is more flexible since it adapts to changing conditions and new data.
- Learning capability: Traditional systems don’t learn or improve over time, while generative AI continuously learns from new data, boosting decision-making capabilities.
- Complexity: Traditional automation is suited for simple and repetitive tasks. On the other hand, generative AI can handle complex decision-making processes that require understanding and predicting outcomes based on patterns in data.
Robotic Process Automation (RPA)
RPA uses software robots to mimic human actions and automate routine tasks across applications and systems. It’s also rule-based and commonly used to automate back-office processes.
- Scope of automation: RPA is limited to only automating repetitive, rule-based tasks. Generative AI can automate a wider range of tasks, including ones that require cognitive capabilities.
- Adaptability: RPA requires manual updates to handle changes in processes or data, while generative AI adapts automatically through continuous learning from new data.
- Intelligence: RPA isn’t capable of making decisions based on data analysis, whereas generative AI can automate tasks and make informed decisions using complex data patterns.
AI-Driven Automation
AI-driven automation uses AI to automate tasks and processes. This includes machine learning (ML) that can analyze data and make decisions based on that analysis. However, not all AI-driven automation has the same capabilities as generative AI.
- Decision-making depth: Standard AI-driven automation can analyze data and make decisions but usually requires significant human input for model adjustments. Generative AI goes a step further by autonomously learning and refining its decision-making processes.
- Predictive capabilities: While AI-driven can predict outcomes based on data, generative AI excels in generating new data and scenarios, providing more comprehensive predictions.
- Application complexity: AI-driven automation is effective for many tasks but struggles to handle highly complex scenarios. Generative AI can handle these situations with high accuracy.
Automated Decision-Making Examples
Industries like healthcare, insurance, and banking have all seen successful implementations of AI solutions for decision automation.
IBM Watson for Oncology
This healthcare example uses AI to assist doctors in making treatment decisions for cancer patients. The system analyzes medical literature, patient data, and clinical trial outcomes to recommend personalized treatment plans. It helps oncologists make informed decisions based on huge amounts of data to improve patient care and treatment outcomes.
Lemonade - AI Jim
Lemonade, an insurance company, uses AI to automate its underwriting and claims processes. Their AI chatbot called “AI Jim” carries out various tasks in minutes, including:
- Interacting with customers
- Processing claims
- Approving payments
This lets them reduce processing time, minimize errors, and boost customer satisfaction by providing accurate responses.
JPMorgan Chase - COiN
JPMorgan Chase uses an AI-powered system called Contract Intelligence (COiN) to automate legal document analysis. It reviews complex contracts and extracts important data points in seconds, typically taking human lawyers many hours. As a result, legal teams can focus on more strategic tasks, such as providing legal advice to support business growth.
Decision Automation in Your Business
Unlike other solutions, AI Agents offer complete automation with minimal human intervention. To find out how your business can implement AI Agents to automate your decision-making workflows, schedule a free 30-minute call with our experts.